
6th Machine Learning and AI in Bio(Chemical) Engineering Conference
06-07th July 2023
In person-only event
Recordings are available!
Machine Learning and AI in Bio(Chemical) Engineering Conference series is co-organised by research groups of the Universities of Cambridge, Leeds, Glasgow, Southampton, and University College London. This series of conferences grew from two collaborative research projects on robotics and automation in chemical development. The conference will feature keynote and invited lectures from global champions of research in ML/AI in chemistry/(bio)chemical engineering, and regular lectures selected from the community submissions.
​
Conference Agenda
The event will be held at the Department of Chemical Engineering and Biotechnology,
Philippa Fawcett Dr, Cambridge CB3 0AS
​
July 06th, day one
10:00 – 11:00 Welcome and refreshments
11:00 – 12:00 Michael Brenner (Keynote) - Scientific uses of automatic differentiation
12:00 – 12:25 Zsuzsanna Koczor-Benda - High-throughput property-driven generative design of functional organic molecules
12:25 – 12:50 Kobi Felton - ML-SAFT: A framework for PCP-SAFT parameter prediction
12:50 – 14:00 Lunch
14:00 – 14:35 Pietro Liò - Generative and Graph models in chemistry and medicine
14:35 – 15:00 Ryan Greenhalgh - Current methods for drug property prediction in the real world
15:00 – 15:25 Egon Heuson - Enzyme activity prediction using neural networks, docking and high-throughput screening results
15:25 – 15:35 Break
15:35 – 16:10 Timur Madzhidov - State-of-the-art on reaction prediction condition
16:10 – 16:35 Benoît Baillif - Applying atomistic neural networks to bias conformer ensembles towards bioactive-like conformations
16:35 – 17:00 Tom Savage - Multi-Fidelity Data-Driven Design and Analysis of Reactor and Tube Simulations (DARTS)
17:00 – 19:00 Networking and dinner
19:00 Day 1 end
July 07th, day two
09:00 – 09:15 Coffee reception
09:15 – 10:00 Workshop part 1 - Rapid predictive modelling without having to write code
10:00 – 10:15 Break
10:15 – 11:00 Workshop part 2 - Rapid predictive modelling without having to write code
11:00 – 12:00 Poster Session
12:00 – 12:35 Antonio Del Rio Chanona - Building Models with Machine Learning
12:35 – 13:00 Jiaru Bai - From Platform to Knowledge Graph: Distributed Self-Driving Laboratories
13:00 – 14:00 Lunch
14:00 – 14:35 Stefan Born - Machine Learning as an integral part of an automated experimental workflow in protein engineering
14:35 – 15:00 Nishanthi Gangadharan - Data-driven Dynamic Control Scheme for Antibody Producing CHO Cell Cultures in Fed Batch
15:00 – 15:20 Break
15:20 – 15:45 Emma King-Smith - Practical machine learning for synthetic chemistry
15:45 – 16:10 Miruna Cretu - Standardizing chemical compounds using language models
16:10 – 16:45 Closing Remarks
17:00 End of day 2
Speakers
We are proud to bring inspirational speakers from across the globe
Confirmed speakers
​
Prof. Pietro Liò (University of Cambridge)
-
Generative and Graph models in chemistry and medicine
​
Dr. Emma King-Smith (University of Cambridge)
-
Practical Machine Learning for Synthetic Chemistry
​
Dr. Ryan Greenhalgh (DeepMirror)
-
Current Methods for Drug Property Prediction in the Real World
​
Egon Heuson (University of Lille)
-
Enzyme activity prediction using neural networks, docking and high-throughput screening results
Timur Madzhidov (Elsevier)
-
State-of-the-art in reaction prediction conditions
​
Benoît Baillif (University of Cambridge)
-
Applying atomistic neural networks to bias conformer ensembles towards bioactive-like conformations
Tom Savage (Imperial College London)
-
Multi-Fidelity Data-Driven Design and Analysis of Reactor and Tube Simulations (DARTS)
​
Nishanthi Gangadharan (University of Cambridge)
-
Data-driven Dynamic Control Scheme for Antibody Producing CHO Cell Cultures in Fed Batch
​
Dr. Zsuzsanna Koczor-Benda (University of Warwick)
-
High-throughput property-driven generative design of functional organic molecules
​
Jiaru Bai (University of Cambridge)
-
From Platform to Knowledge Graph: Distributed Self-Driving Laboratories
Miruna Cretu (IBM Research Zurich)
-
Standardizing chemical compounds using language models
Kobi Felton (University of Cambridge)
-
ML-SAFT: A framework for PCP-SAFT parameter prediction
Dr. Antonio Del Rio Chanona (Imperial College of London)
-
Building Models with Machine Learning
​
Dr. Stefan Born (Technische Universitaet Berlin)
- Machine Learning as an integral part of an automated experimental workflow in protein engineering
​
Dr. Michael P. Brenner (Harvard University)
-
Keynote talk: Scientific uses of automatic differentiation
Workshop
Rapid Predictive Modeling without Having to Write Code
Volker Kraft, JMP Principal Academic Ambassador
Phil Kay, JMP Learning Manager
Keynote speaker for 2023 edition
Dr. Michael Brenner (Harvard)
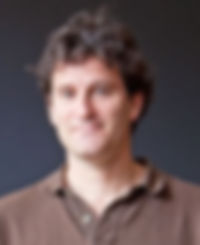
Workshop

Rapid Predictive Modeling without Having to Write Code
Volker Kraft, PhD, JMP Principal Academic Ambassador
Phil Kay, PhD, JMP Learning Manager
​
JMP Pro is an industry-standard software for predictive modeling. In fields like chemistry & biotech, pharma, manufacturing or environment & sustainability, modern modeling methods and powerful tools help engineers and scientists to make statistical discoveries from data – without the need to write any line of code.
​
During this hands-on workshop you will learn how to:
-
build better and more useful models with modern predictive modeling techniques, such as regression, neural networks, decision trees, support vector machines, k nearest neighbors, and more;
-
fit multiple models automatically and select the best-performing model with model screening;
-
apply cross-validation techniques to avoid overfitting;
-
use the Prediction Profiler to improve model understanding and make predictions;
-
deploy models both inside and outside of JMP (e.g., in Python)
Abstract Submission
The 6th International Conference on Machine Learning and AI in (bio)Chemical Engineering will take place on July 06-07, 2023. We are requesting abstracts for posters and talks related to this theme. Potential topics/submissions include but are not limited to:
​
-
Software implementations of key methods
-
Experimental case studies using ML
-
Method development, particularly those that simplify user experience
-
Benchmarking of ML methods
Abstracts may be up to 400 words and optionally include explanatory figures. We emphasise that abstracts should include sufficient introduction for newcomers.
Registration
06-07th July 2023
Hosted by the University of Cambridge
Standard registration: £75
Fees include access to the event, refreshments for both days and confererence networking dinner in the evening of day 1.
​
Payments can only be done by credit card.